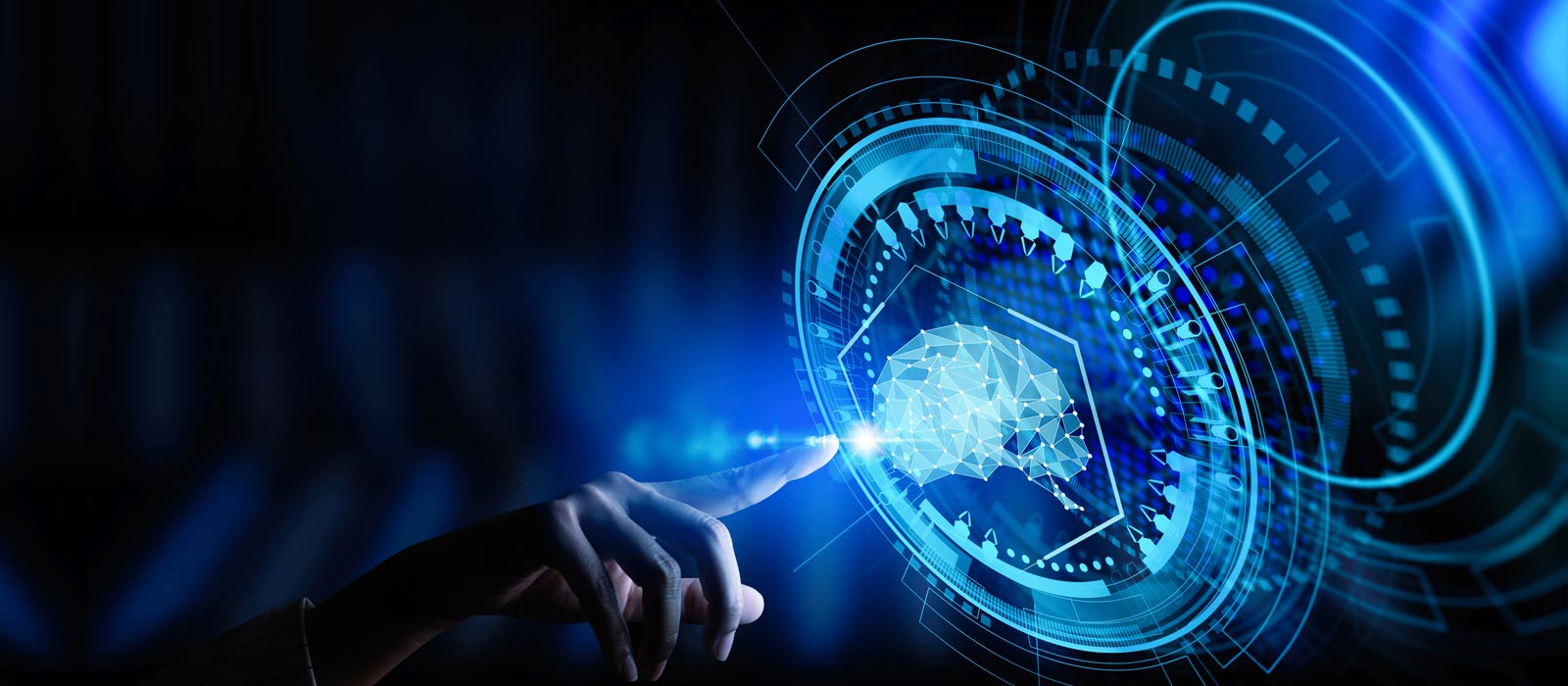
26 May Deep Learning and Indoor Positioning Technologies
Most of us have probably experienced this before: you’re inside a large structure, such as a shopping mall, event center, or underground carpark and your navigation system has trouble locating you on the map. This is usually caused by the degradation of your GPS signal caused by the concrete walls of the structure. Your smartphone apps can use your location to provide contextual information. You may use that information to get driving directions, find a store, or receive alerts for nearby promotions. These handy features are enabled by GPS, which requires outdoor exposure for optimum accuracy. However, inside large structures you might have difficulty accessing this information due to a poor GPS signal.
Accurate indoor positioning systems (IPS), based on public sensors and user permission, can provide some level of location-based information even when you are not outside. An (IPS) is a network of devices used to locate people or objects where GPS and other satellite technologies lack precision or fail entirely. This can include multistory buildings, airports, alleys, parking garages, and underground locations. Current IPS solutions have poor accuracy, especially in multi-level buildings. This article outlines a summary of the currently available IPS technologies.
Indoor Positioning Technologies in Brief
Bluetooth Low Energy (BLE) technology can detect the general location of a person or object enabling continuous asset tracking with at least room-accurate location via BLE sensors/beacons. Position calculation using the Angle of Arrival (AoA) allows much more precise localizations but comes with high sensor infrastructure support and hardware costs. BLE and Beacons have emerged as the ideal indoor location technology considering the lower costs and ease of application.
WiFi based systems use WiFi transmitters as tags to send readings to several WiFi access points. Information algorithms use those readings to compute the position of the source. The location information is eventually sent to a cloud environment. Systems using WiFi and ‘time difference of arrival’ (TDOA) technologies give a fairly high level of accuracy (within 3 to 5 meters) but these systems can be expensive.
UWB Ultra-Wideband (UWB) systems achieve very high accuracy via three-dimensional positioning. Continuous, highly accurate, asset tracking is possible due to the extremely wide UWB signal and the capability to transmit a very wide pulse over a GHz of spectrum. Historically, systems based on UWB have achieved the highest accuracy. Even though UWB tags are inexpensive, every location must have at least three readers due to the confined ranges of the tags. This makes a UWB solution significantly more expensive that a BLE solution.
New Deep Model Solutions
By applying algorithms or deep models, data coming from these sensors can be optimized to improve speed estimation, noise reduction, zero-velocity detection, and altitude – position prediction. One of the main problems in the navigation field is speed estimation. As the estimation becomes more accurate, it will also affect the position solution. Low-cost sensors suffer from high levels of noise and are characterized by a noise profile that changes with time. Use of an appropriate filter can help reduce the noise. As these noise profiles are difficult to estimate, the use of deep learning algorithms can help in analyzing, predicting, and correcting these profiles.
Technology-fueled IPS can be applied to industries such as manufacturing, retail, automotive, field service, and more. The exact indoor positioning of assets in a building enables the optimization of internal logistic processes as well as staff management and can therefore be a valuable tool for increasing efficiency while reducing costs.