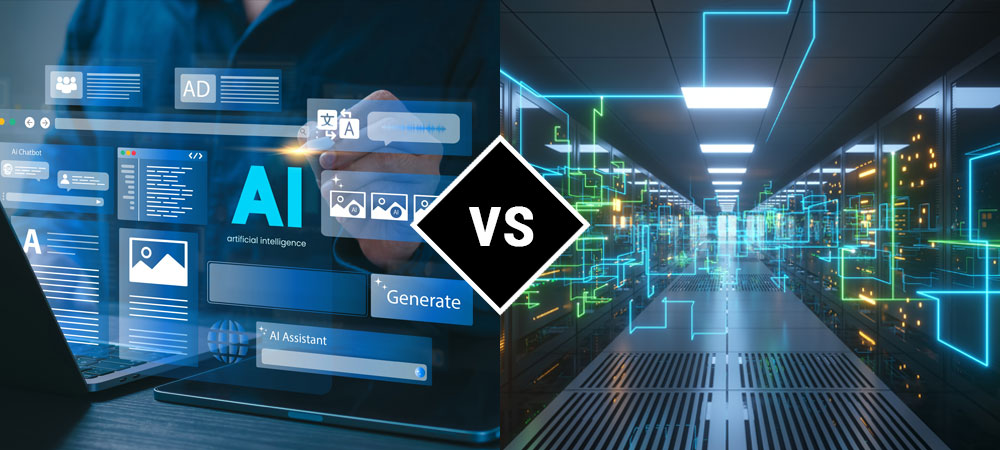
07 Oct GenAI vs AI – Understanding the Differences
Artificial Intelligence is reshaping the ways in which companies operate and perform. Around the globe, traditional AI and machine leaning are revolutionizing the performance, security and sustainability of machines, networks, and processes across every industry. Companies in manufacturing, telecommunications, oil and gas, automotive, and utility management are leveraging AI-enabled products and solutions as integral elements within their Industry 4.0 initiatives.
Where does Generative AI (GenAI) fit into all of this, and how does GenAI differ from traditional AI?
GenAI – Augmenting the Benefits of Traditional AI
While GenAI is a subset of traditional AI, there are distinct differences between the two in terms of definition, purpose, capabilities, and applications.
- AI refers to the broad field of computer science aimed at creating machines capable of mimicking human intelligence. This can range from simple rule-based systems to complex decision-making algorithms. Traditional AI systems typically follow pre-programmed logic or learn from data to make predictions or decisions, often based on identification of baseline patterns and deviations from those patterns.
- Generative AI, a subset of AI, focuses on creating new data rather than just analyzing or predicting outcomes from existing data. It uses machine learning models, often based on deep learning techniques like neural networks, to generate content. These models are trained to create new, human-like data by learning the underlying patterns from large datasets.
Application and Functionality
- AI is used to analyze, classify, predict, and automate tasks. Its core function is decision-making based on the collection and analysis of machine, network, and process-generated data. For example, AI can power recommendation systems, fraud detection tools, self-learning machines, and intelligent workflows. Traditional AI excels at learning patterns and using them to improve performance, reliability, and security.
- GenAI specializes in creativity. GenAI solutions are used to generate new outputs such as complex models, images, text, predictive insights, and more. Rather than just analyzing existing data for insights, generative AI creates new data that provides forward-looking operational improvement.
GenAI can be thought of as an aggregation engine for all the points of intelligence created within a traditional AI ecosystem. While traditional AI can provide insights and model-based predictions it does not really create anything new within the ecosystem. GenAI does. By aggregating and analyzing the various inputs of AI and machine learning you can query GenAI for recommendations for new solutions and to provide faster insights into current conditions. For example….
- Why did machine B outperform machine A during a given period?
- What are some recommendations for bringing machine B up to machine A’s level of performance?
- What is causing our shift over shift variances in product quality?
- How can I improve OEE for a single machine and/or across a group of machines?
Core Technologies
AI incorporates a variety of advanced technologies, including:
- Machine Learning (ML): This involves training models to recognize patterns in data and make predictions.
- Deep Learning (DL): A subset of ML, deep learning utilizes neural networks to process vast amounts of data and to generate real-time insights from that information.
- Natural Language Processing (NLP): A field of AI focusing on understanding and generating human language.
GenAI, while relying on some of the same technologies, focuses more on:
- Generative Adversarial Networks (GANs): GANs are a class of machine learning frameworks where two neural networks compete against each other to create realistic data or content.
- Transformer Models: These models, like GPT or BERT, are commonly used for generating language or images and code.
- Variational Autoencoders (VAEs): Are used to generate new data by learning a compressed representation of input data and then decoding it to produce new content.
GenAI-Empowered Knowledge Management
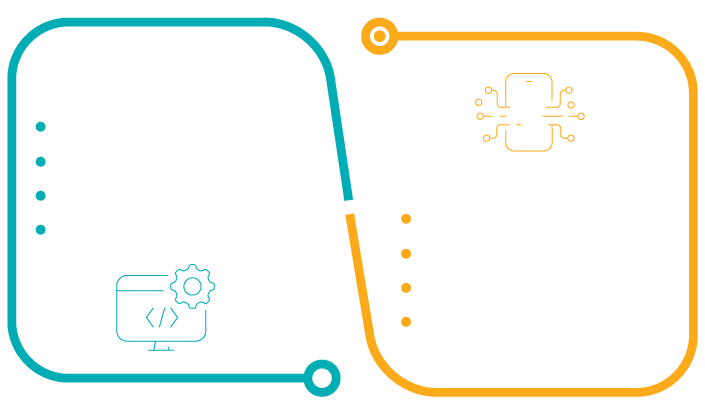
Both AI and GenAI are powerful technologies, each with its unique strengths and applications. While traditional AI excels in automating tasks and analyzing data, generative AI pushes the boundaries of creativity by producing new content and predictive insights. As these technologies evolve, they will intersect in more ways, allowing for enhanced decision-making and operational optimization. Understanding their differences can help organizations and individuals leverage them more effectively in their respective fields.