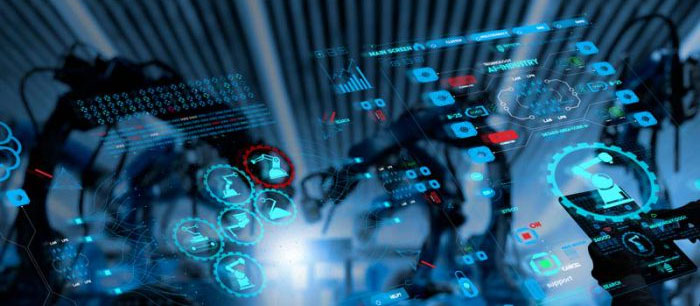
16 Dec Predictive Manufacturing – Advantages at the Extreme Edge
Manufacturing operations are constantly looking for ways to improve output, efficiency and quality, while also reducing their COGS. It had become increasingly difficult to find new methods and technologies that would continue to deliver measurable and sustainable operational improvements within a manufacturing environment. The advent of predictive manufacturing has changed that. Manufacturers now have a powerful Edge-native AI tool at their disposal that can deliver new levels of operational excellence and OEE (Overall Equipment Effectiveness).
Manufacturing Challenges
Regardless of the types of products being produced, companies within the manufacturing segment face many of the same challenges and obstacles. Many operations are struggling to close an intelligence gap that blocks the path to predictive manufacturing. Those obstacles include the following:
- Lack of intimate, asset-centric, machine performance data: The absence of Endpoint AI embedded and trained at the asset microcontroller (MCU) level restricts the ability to attain asset-specific insights and to develop predictive maintenance models. This creates blind spots within the manufacturing ecosystem.
- Costly, unplanned, machine or device downtime: Continued reliance on legacy preventive maintenance processes that are unable to self-adjust based on current real-time conditions of the asset. This often results in maintenance that is performed too early or too late.
- Expensive and inefficient asset data collection: No ability to collect, synthesize and analyze machine data locally severely restricts the ability to automatically generate alerts and corrective actions. Assets often perform at suboptimal levels for extended periods of time.
- Exposure of critical data to cyber-attack: Traditional cloud-based AI solutions can expose critical asset data during the process of transferring and storing that data within the cloud. This has proven to be a prime breeding ground for malicious cyber-attack.
The Solution
Manufacturers now have the option to embed AI and machine learning (ML) at the extreme edge. This means that AI and ML algorithms can now be embedded and trained directly into an asset’s MCU. This Edge-native AI technology is the foundation for the transformation to predictive manufacturing. The pillars of this technology include:
- Endpoint intelligence: AI and ML embedded directly into an asset’s MCU – AI at the edge of the edge.
- Asset-specific intelligence: Customization of AI enablement parameters to accommodate the requirements of specific machines and devices.
- Self-learning algorithms: Intelligence that learns and evolves to provide optimized asset performance and predictive maintenance.
- Intelligent, automated, workflows: Edge-AI enabled workflow that deliver real-time alerts and generate corrective actions.
- Enhanced cyber-security: Less reliance on cloud infrastructures = less exposure of critical data to cyber criminals.
The Impact
The move from reactive to predictive manufacturing is producing a transformational impact for many companies in the manufacturing sector. Companies are reporting improvements in several operational areas. A sampling of those would include:
- Improved OEE: Many companies are increasing OEE levels to 85% or higher.
- Predictive maintenance: Reduction in unplanned/unnecessary downtimes improves asset productivity and ROI.
- Maintenance efficiency: Predictive maintenance improves the utilization of maintenance staff and reduces overhead.
- Asset heath and performance: Endpoint AI delivers faster identification and remediation of performance issues and improves asset health and longevity.
- Secure assets and data: Assets and their data are controlled locally, significantly reducing expose to cyber-attack (including Zero-Day attack).
- All the above combine to improve production capacity, resource utilization, waste control, asset lifespans, and profit margins.