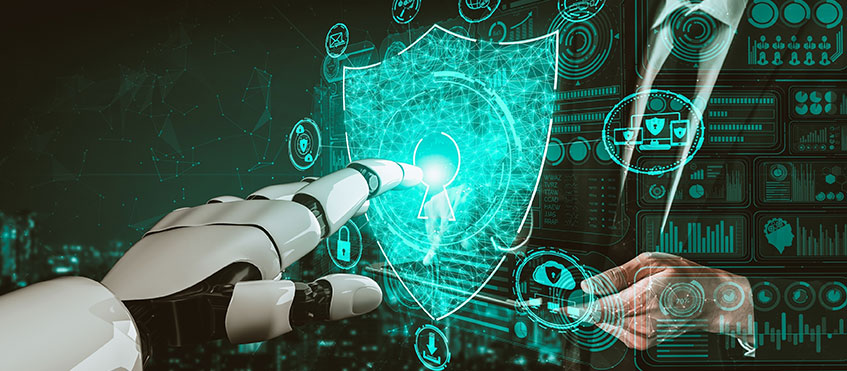
09 Jun Moving AI From the Cloud to the Machine Endpoint
The manufacturing and industrial automation segments are continuously adopting new methods and technologies to improve the performance of their production environments. Maximizing the health, output, and security of machines and devices can have a dramatic impact on overall equipment effectiveness (OEE), fueling improvements in cost, quality, and competitiveness.
Over the past decade, many factory floors have integrated cloud-based AI solutions to help better manage IIoT devices and machines. While those solutions have helped bridge the gap between automation and intelligence, they do have limitations. Moving intelligence from the cloud to the extreme endpoint will be the next major Industry 4.0 initiative.
The Challenge – Faster and More Secure Intelligence
Manufacturers that rely on legacy cloud-dependent AI solutions are now facing new operational challenges that will require asset intelligence that is much more asset-centric. Those challenges include, but are not limited to, the following:
- Cyber-security: Cloud-based solutions require exposure of sensitive data during transmission and storage, thereby increasing the risk to cyber-attack.
- Disparate data processing routines: Inconsistent processing of machine performance data creates gaps in visibility and prevents behavioral modifications
- Requirement for labeled data: Most legacy cloud-based AI solutions are heavily dependent on data that has been thoroughly cleaned and labeled, creating additional process steps and cost.
- Delayed data processing and reaction time: Data latency is an inherent issue when processing data via a cloud-based architecture, resulting in delay in delivery of critical insights.
- Limited insights into specific machine performance: Manufacturers lack the ability to embed intelligence directly into their mission-critical machines and devices, preventing the asset-specific control that is needed within today’s manufacturing environments.
The Solution – Intelligence at the Endpoint
New Edge-native AI solutions will completely transform the use of AI and machine learning within the manufacturing environment. Endpoint AI technology will provide manufacturers with the means to embed intelligence directly into their most critical assets. Key features of these solutions will include:
- Machine-centric intelligence: AI and ML algorithms are embedded and trained within the machine’s microcontroller (MCU), providing ultimate customization of intelligence.
- Elimination of labeled data: Edge-native AI solutions will not require the use of labeled data. More cost and process effective.
- Local control: All critical machine data is collected, processed, and analyzed locally, eliminating the need for external systems and cloud architectures.
- Self-contained analytics: Smart analytics built directly into the individual device or machine provide real-time reporting and alerts.
- Tangible endpoint agent: Asset-specific intelligence that actively learns and reacts to abnormal performance and/or security alerts.
The Impact
Moving from the cloud to the endpoint will revolutionize the factory floor in several ways, including:
- More robust security: Manufacturing environments are high-value targets for cyber criminals. Reducing the amount of data that is exposed to external environments limits a hacker’s ability to access that data. Machines, devices, and data are much more secure.
- Predictive manufacturing: Manufacturers will have the level of AI-enabled intelligence to move many of their processes into a predictive state. This will include asset-specific predictive maintenance.
- Improved overall equipment effectiveness OEE: Edge-native AI provides the means to improve OEE performance from the current standard of 70% to a new standard of ~ 85%.
- Enhanced cost control and improved quality: Intelligence at the endpoint will provide the means to reduce infrastructure costs while also providing the real-time intelligence needed to improve product quality.