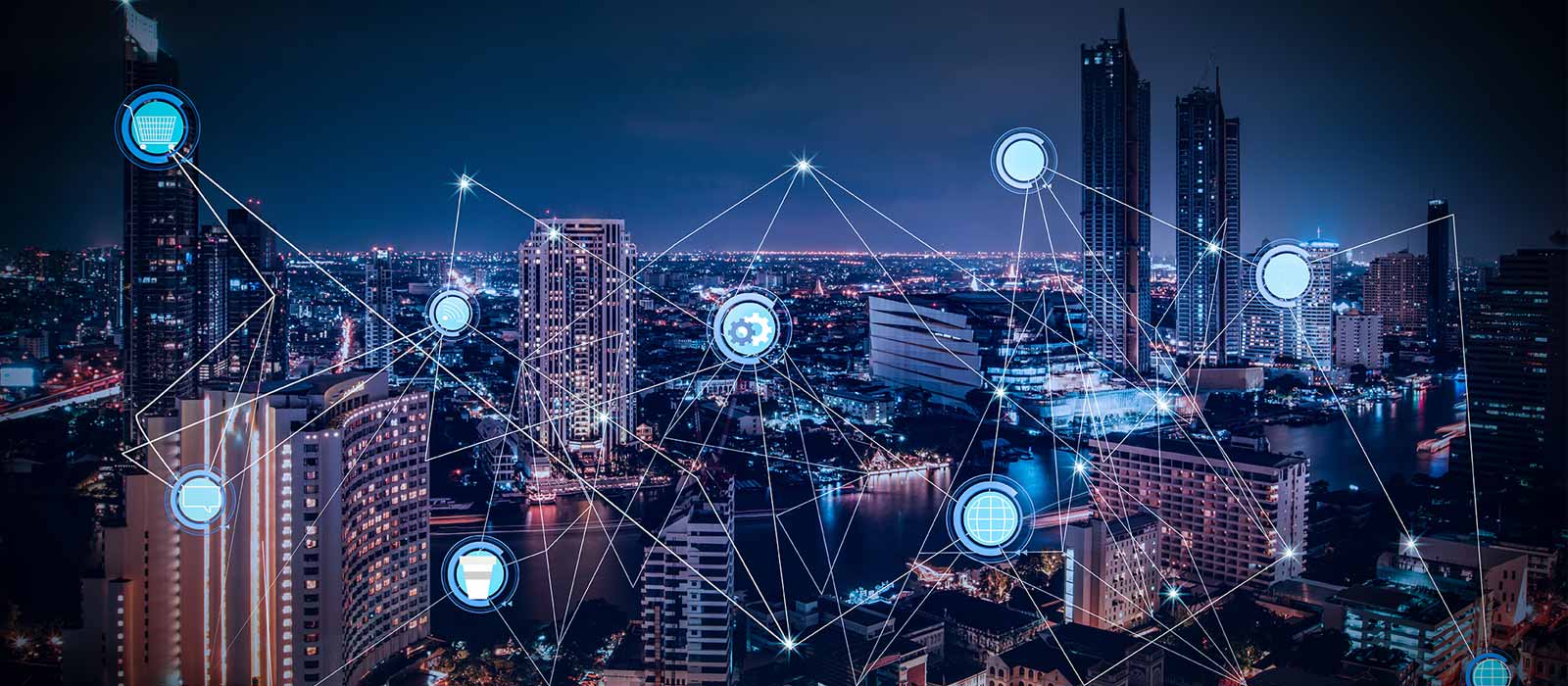
02 Sep Endpoint AI – Solving Telecom Business Challenges
Endpoint AI – Solving Telecom Business Challenges
Despite ongoing consolidation, competition within the telecom segment is at an all-time high. With economic, social, and legislative factors helping to provide more level playing fields, telecom companies are now faced with increased pressure to find new pathways to differentiation. The search for competitive distinction now involves all elements of the telecom value chain (operations, services, support, etc.).
The Industry Challenges
Telecom operators share a few common challenges within today’s evolving business environment. While by no means a comprehensive list, those issues would include the following:
- A Lack of deep performance insights: The lack of integrated, telecom device and network centric, Endpoint AI solutions makes it difficult for operators and device OEMs to develop the real-time performance insights that would bring new value to their customers.
- Collection and aggregation of device analytics: In many cases, telecom operators have no ability to automate the collection, analysis, and visualization on network devices that share a common IMEI TAC (International Mobile Equip Identity – Type Allocation Code).
- Network, device, and data security: As the volume of connected devices continues to accelerate, so does the need to provide enhanced cyber-protection of those devices and the data they produce. Network operators are challenged to stay ahead of today’s sophisticated cyber-criminal.
The Solution
Moving embedded artificial intelligence (AI) and machine learning (ML) much closer to the telecom network, and its enabled devices, provides the foundation for delivery of “smarter” telecom solutions. Endpoint AI (AI at the extreme edge) represents a fundamental shift in the way that AI and ML algorithms are embedded and trained.
MicroAI AtomML™ embeds intelligence directly into the microcontroller (MCU) of a smart device or network component. There are several transformational advantages of this new approach, including:
- Speed of insights: Data collected at the device or component level is collected, analyzed, and acted upon much faster than is possible with traditional AI systems.
- Superior integration: AI and ML embedded into the network and integrated with smart device data improves the performance of the entire telecom ecosystem.
- Lower cost: Endpoint AI reduces or eliminates the dependance on cloud infrastructures for data processing and storage. This significantly reduces AI and ML operational costs.
The Impact
The impact of MicroAI AtomML™ will be transformational and will include:
- Enhanced network capabilities: The input of AL and ML algorithms closer to critical network components enables the use of real-time predictive analytics that improve network performance and reduce costs.
- Deeper device insights: The ability for telecom operators to transmit device performance data back to device OEMs and customers. A closed-loop system that improves overall device/network performance.
- Endpoint-AI security: Self-learning security protocols embedded at the network and device levels deliver state-of-the-art security to operators, OEMs, and customers.
- Improved customer satisfaction: Quality of Service (QoS) metrics generated by AI-enabled analytics will power the fine-tuning of the customer experience and help build brand loyalty.