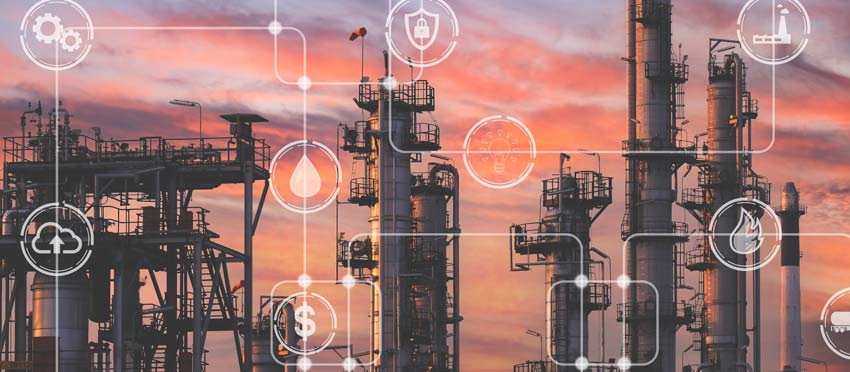
23 Dec Enabling Predictive Maintenance in Energy Production
The harvesting and processing of energy resources requires efficient management of several complex and costly processes. Whether it be oil or natural gas, these processes depend on reliable—and predictable—performance from a wide variety of IIoT (industrial internet of things) devices and machines. These assets perform critical functions within every phase of the energy harvesting, refinement, storage and delivery ecosystem.
Historically, the energy sector has relied on preventive maintenance routines to help keep production assets performing safely and reliably. However, preventive maintenance processes have inherent limitations. To reach higher levels of Overall Equipment Effectiveness (OEE), improved cost control, and enhanced safety, an innovative approach was needed.
The Problem
The transition from reactive to preventive maintenance a few decades ago provided the energy sector with much-needed improvement in the management of mission-critical assets. Technology is never static and what was considered cutting-edge then is considered outdated today. As the competitive and regulative landscapes have changed, the shortcomings of legacy preventive maintenance solutions have become painfully apparent. Shortcomings that include:
- Lack of actionable, real-time, asset intelligence: Legacy preventive maintenance processes are based entirely on historical data. History is not always an accurate predictor of current and future conditions. This often creates a wide intelligence gap.
- Time-based instead of intelligence-based maintenance: Maintenance schedules that are based on non-intelligent timetables that are not connected to real time data. Device and machines are often shut down to early (unnecessary production stoppage) or too late (damage to asset).
- Inability to predict emergency situations: No ability to predict the potential for catastrophic failure due to lack of AI-enabled, asset-specific, predictive analytics. This creates increased potential for an emergency at the asset and/or operational level.
- Static and manual maintenance routines: The reliance on manual maintenance processes that consume human resources and that are prone to error. Maintenance routines that are static and difficult to adjust.
- Non-AI enabled safety protocols: Outdated asset protection processes that are not supported by AI-enabled, self-learning, fault-detection and alert capabilities. No ability to predict potential failure and safety issues.
The Solution – Predictive Maintenance
To address today’s challenges, the energy sector is moving from static preventive maintenance routines to predictive capabilities fueled by current advancements in Edge-native AI technologies. MicroAI AtomML™ is the latest AI and ML (machine learning) platform that lives directly on the microcontroller (MCU) or microprocessor (MPU) of an IIoT device or machine. MicroAI AtomML drives the enablement of predictive maintenance capabilities by:
- Embedding and training AL and ML algorithms directly onto the MCU or MPU of the device or machine. This can include pumps, sensors, valves, engines, compressors, flow controllers, etc. Edge AI that is customized for the specific asset type.
- The AI and ML algorithms learn the normal behavior patterns of the asset under various operational conditions. This self-learning occurs with no requirement for human intervention.
- Real-time, asset-specific, performance data is automatically collected and analyzed to produce predictive maintenance intelligence based on current and projected asset health and performance. Maintenance that is based on dynamic asset data vs static schedules.
The Impact
Edge-native AI predictive maintenance will allow companies in the energy sector to maximize the performance, safety, and security of devices and machines throughout the entire energy value chain. Positive impacts will include:
- Asset-centric maintenance: Predictive maintenance based on the current and projected performance of an asset. Maintenance based on real-time data vs fixed routines.
- Increased asset uptime: Elimination of asset downtime due to maintenance that may not be required. More efficient utilization of maintenance staff resulting from predictive data.
- Catastrophic failure avoidance: Local, real-time, predictive analytics that detect and alert on the possibility of device or machine failure. Intelligent, automated, workflows that help ensure rapid response and mitigation.
- Enhanced Safety: Predictive maintenance helps improve asset and human safety throughout the entire energy production lifecycle.
- Improved OEE: The energy sector has traditionally reported OEE scores in the low 70’s. The transition to AI-enabled predictive maintenance can help reach scores of 85% or higher.