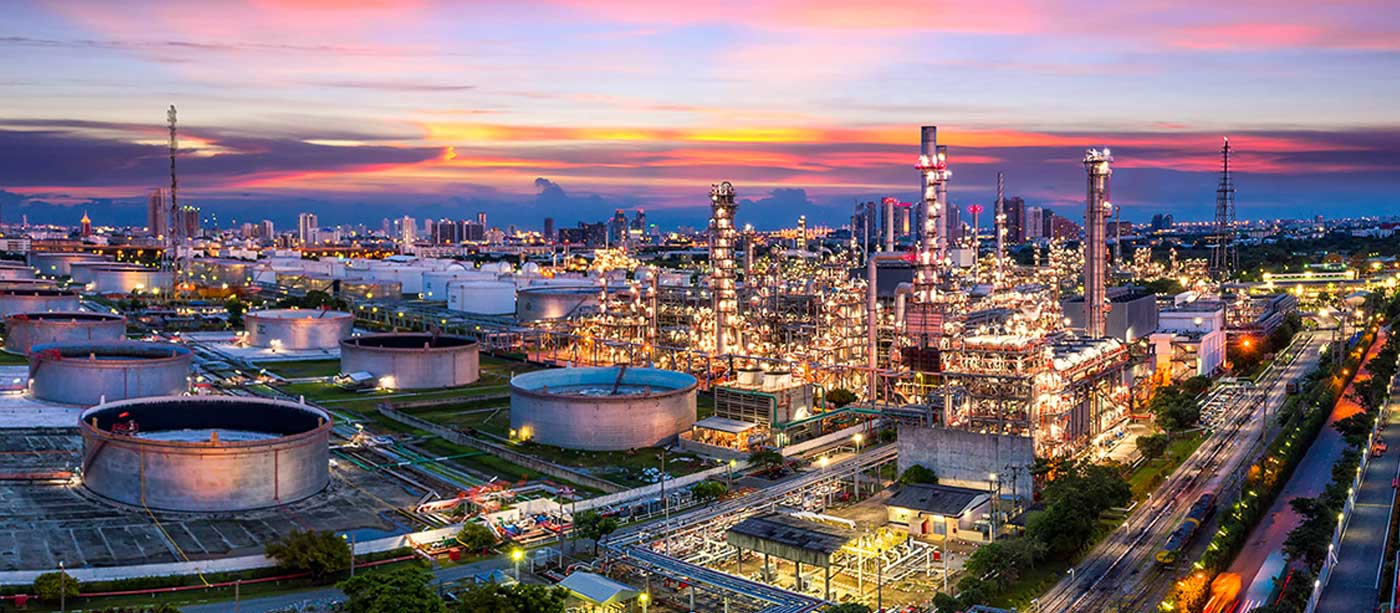
16 Sep Edge-native AI – Revolutionizing the Oil & Gas Industry
Operators and service providers within the oil and gas industry are finding it increasingly difficult to establish competitive advantages when hampered by the use of outdated technology solutions and architectures. Continued reliance on mission-critical assets that are non-optimized, disconnected, and vulnerable to cyber-attack can produce operational blind spots, production bottlenecks, and security breaches that create higher costs and degraded performance. To address these challenges, many operators are looking to artificial intelligence (AI) and machine learning (ML) solutions as a pathway to true operational excellence.
New approaches to the delivery of AI/ML models now make it possible to create an AI ecosystem that is local and asset centric. Edge-native AI (AI at the extreme edge) represents a paradigm shift in the way that intelligence is delivered and modeled as well as the process for data analysis and storage.
Operational Challenges
For most of us the process of producing energy appears simple and straightforward: locate the oil/gas, get it out of the ground, process it, ship it. The reality is much more complex. Oil & gas operational challenges can be broken down into four basic categories.
Asset Optimization: Oil & gas companies are asset intensive. Those assets represent large investments in capital and their performance are critical to overall operational performance. A sampling of challenges would include:
- Predictive maintenance: The ability to accurately predict when critical assets will require maintenance is a prime objective for all operators. Benefits include reduced downtimes, longer asset lifespans, and more efficient use of maintenance resources.
- Drilling process optimization: Automation, accuracy, and repeatability all combine to dictate the level of efficiency of the drilling process. Workflows that learn and evolve (intelligent workflows) are needed to go beyond traditional process automation capabilities.
- Anomaly detection: Too often a performance anomaly, or fault, is not detected until significant damage to an asset or its productivity has already been done. This can include pump performance, system pressure, hotspot detection, container integrity, etc.
Data Analysis and Insights: The ability to quickly react to changing conditions—in real time—can be the difference between operational excellence and sub-par performance. Problems can include:
- Well performance ranking: The lack of well-specific insights makes it difficult to rank individual well performance in real time. This has a negative impact on overall production efficiency.
- Pump and Engine Analysis: Post break-down reaction to pump and engine failure creates bottlenecks in the production process. More intimate real-time analytics are needed.
- Quick Fault Analysis: Identifying root-cause of failure is difficult due to the pressure to resume operation. Endemic problems are often not detected.
Safety: Reliable compliance with OSHA safety regulations must be top-of-mind for any oil & gas operation. Common challenges include:
- Emergency shutdown prediction: Operators need to be able to predict the advent of an emergency shutdown situation. This requires the embedding of intelligence at the critical asset level.
- Tank fault detection: Detection of tank faults (leaks, degradation, etc.) often come too late to prevent loss of integrity and spillage. A more predictive capability is needed.
- Hot-spot detection: Hot spots are common in the energy production process. Real-time detection and remediation are critical. Reducing the dependance on human intervention has been difficult.
Security: Cyber-threats are getting more sophisticated and more destructive. The oil & gas industry can be a prime target for cyber-criminals. Today’s security challenges would include:
- Data exposure: Many operations utilize a cloud environment for the processing and storage of asset and operational data. This can expose critical data to cyber-attack.
- Asset vulnerability: Many mission-critical assets are vulnerable to cyber-attack due to a lack of asset-centric security protocols. Attacks are not detected until the assets have been fully compromised.
- Lack of predictive insights: No ability to predict asset and data vulnerability. Cyber-security measures are purely reactive and typically not granular enough to address localized threats.
Edge-native AI for Oil and Gas
Meeting the challenges highlighted above will require the transition from reactive to predictive operational management. This, in turn, will require the embedding of AI and ML as close as possible to the critical assets within the oil and gas operation.
MicroAI AtomML, MicroAI Insight, and MicroAI Security will provide the oil and gas industry with a complete suite of Edge-native AI products to meet the challenges of today and tomorrow.
The Basics
- Local intelligence: AI is embedded and trained onto individual assets (AI at the extreme edge). This brings big infrastructure intelligence down to the asset MCU/MPU level.
- Asset-centric insights: Real-time insights from relevant data sources including sensor and telemetry assets (SCADA, DCS, PLC, RTU, etc.). Local processing of data significantly improves response times.
- Predictive maintenance: Embedded AI algorithms that produce predictive insights to optimize maintenance schedules, reduce downtimes, and control maintenance costs.
- Enhanced cyber-security: Local, non-cloud-dependent, security monitoring to protect critical assets from cyber-attack—proactive protection of assets and the data they produce.
Impact to Operational KPIs
Adoption of Edge-Native AI will have significant impact on KPIs within the oil and gas segment.
- Reduction in asset failure rates
- Increase Mean Time Between Failure (MTBF)
- Reduction in field service costs
- Reduced maintenance downtimes via predictive maintenance
- Increased lifespan of high-value assets
- More predictable and reliable production outputs
- Process accuracy and predictability via intelligent workflows
- Enhanced security—real-time detection/prediction of cyber-threats
- Reduction in overall operational costs
- Improved customer satisfaction and brand loyalty