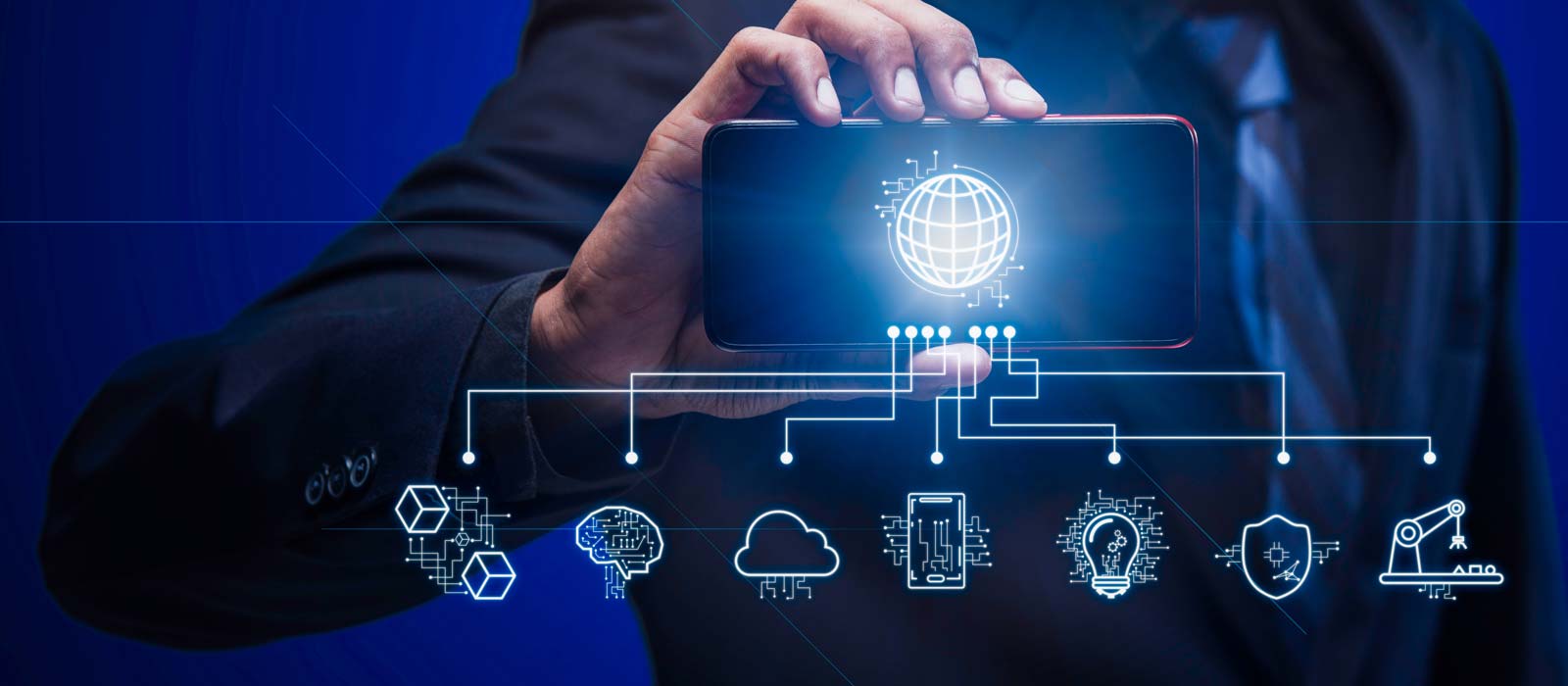
17 Jun Edge AI – Maximizing the Performance of Your IoT Deployment
Industrial Internet of Things (IIoT), Industry 4.0, and AI-powered solutions are no longer “futuristic” ideas. There are real IIoT deployments in production that are generating a massive amount of value and return on investment for organizations across the globe. Along with the value that is being created, there is also a tremendous amount of data being generated. How organizations manage, store, process and act upon the data that is produced is what will determine if a deployment is merely successful or if it reaches the level of being transformational for the organization.
Capturing Industrial Sensor Data
Industrial IoT deployments usually focus on providing insight into equipment and other company assets. That limited focus tends to provide little transparency into how the assets are performing or how they are being utilized. To accomplish this, generally data is extracted by one of, or a combination of, these two methods:
- Exploit existing intelligence that already exists within the asset (i.e. built-in sensors from the OEM) – This is more common with newer equipment as OEM’s are becoming more advanced with embedding sensor technology within their equipment. Some of these built-in sensors can pull at a rate of 1 data point per 100 milliseconds. This can provide very granular insight into the machine.
- Additional sensor placement – For legacy or older equipment that has limited to no existing intelligence, it is common practice to place additional sensors onto the asset to understand its behavior. These sensors can range from bearing temperature probes and acoustic emission sensors, to accelerometers and gyroscopic sensors, along with many more.
Capturing this data is merely the first step in a 3-step process for deploying a transformational IIoT solution. (1. Data Capture | 2. Data Processing | 3. Triggering of Action based on processed data output- Ideally, automated action and events). Each asset now has sensor data being generated and captured. The next step is what to do with this data.
For example, if a deployment has only 10 assets being monitored, and each asset has 10 unique sensor values being generated every second, over the course of a single hour, 360,000 data points are being generated. If this solution were to scale to hundreds of assets and data is pulled at a faster rate than once per second, millions of data points are generated daily. If these connected assets are remote and a cellular or satellite communication protocol needs to be used, the cost to transmit this data would be very high. Additionally, once this data is received, the cost to store and process this data in a cloud infrastructure would add up quickly as well.
Process Data at the Edge
Unleashing the power of Edge AI. You can now see that a vast amount of data is being generated at the edge from these connected assets, and that shipping data and storing data within a cloud infrastructure could be cost prohibitive. This is why MicroAI has created MicroAI™– an Edge AI product that is designed to live in low-cost, small footprint, low compute power devices that sit at the edge to process data locally.
The use of MicroAI™ allows for massive amounts of data to be captured and analyzed without the need of shipping across costly network communication protocols, or storing in expensive cloud infrastructure. Only processed data, specifically alerts and notifications created via the MicroAI™ behavioral analysis algorithm, are triggered to be sent to key stakeholders to take corrective action.
Furthermore, Industry 4.0 solutions should be predictive and automated. Hence why MicroAI™ detects anomalies, signs of failure and possible issues with the equipment and immediately triggers action to mitigate the risk of unexpected downtime. This action component of MicroAI™ can range from sending a simple email to the asset owner or calling an API to open an automated workorder for tracking of the ticket through to completion. It can also include activating a change within the machine cycle to lower the risk of an issue (i.e. decreasing RPM/cycle rate to reduce strain on a bearing that is showing signs of wear).
Training at the Edge
MicroAI™ is designed to train at the edge. This is unique within the market. Most vendors within the space are deploying AI models that are trained in a cloud environment and pushed down to the edge locations. This means that each asset out in the field utilizes the same model. This may be satisfactory to some level, but no two assets are under the same conditions. The assets may be in various geographic locations with much different environmental pressures, or the same make and model of an asset may be preforming unique tasks from one another. This is why MicroAI™ is was built and designed to train and process data at the edge.
Edge AI is a must have when considering an Industrial IoT deployment.