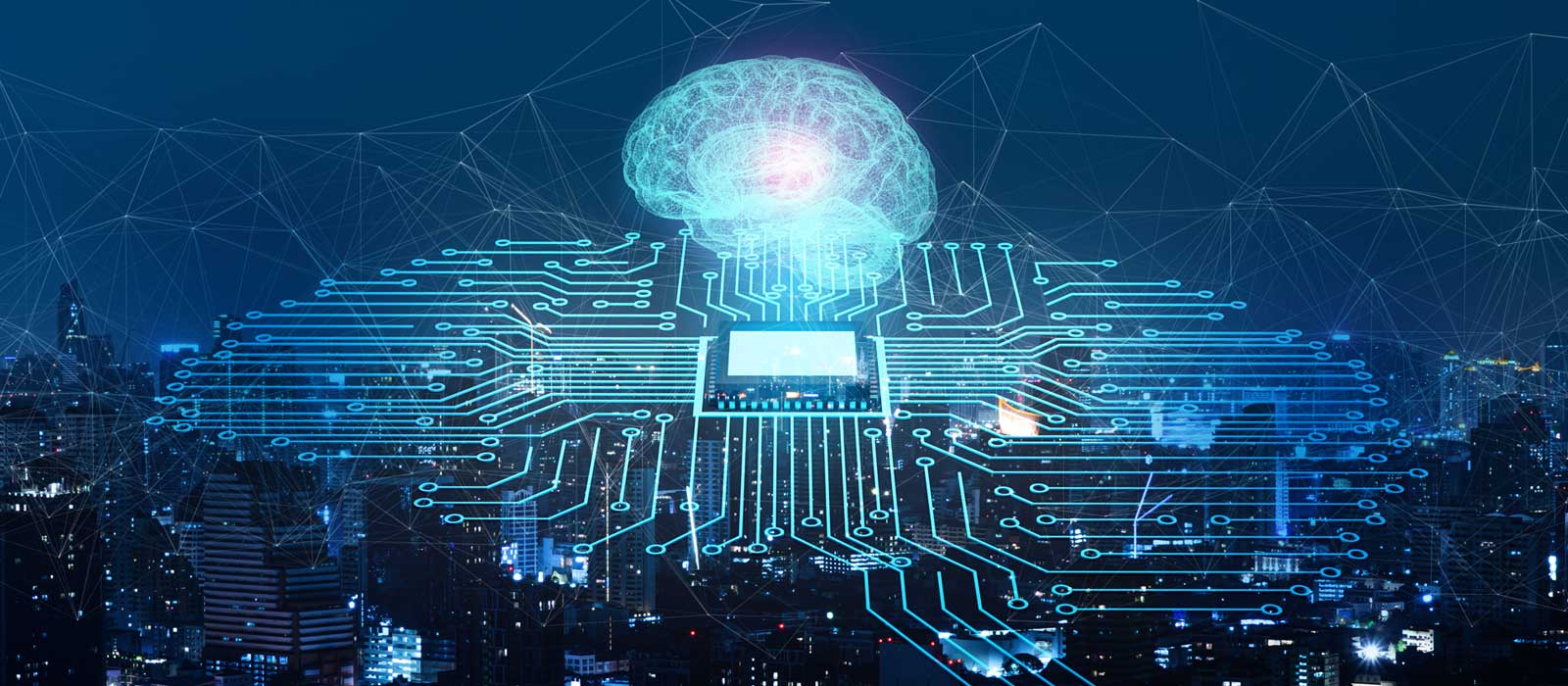
30 Sep Edge AI – A More Local and Secure Approach to AI
The ability to access and analyze real-time data is critical for any business enterprise. Advancements in AI-enabled data collection and analysis have provided significant computing benefits which fuel improvements in operational performance.
For traditional AI solutions, the processing and storage of data is executed via a cloud computing architecture. All data is processed in a centralized data center and managed and maintained by cloud service providers. Connections to the cloud environment can be fixed (ethernet, cable, or fiber optic) or by way of a radio frequency (RF) connection that can come in many configurations including 2G, 3G, 4G, LTE, 5G, WiFi, Bluetooth or new low powered wide area network (LPWA) like LoRaWAN.
The cloud-based approach provided the baseline for operations looking to leverage the power of AI and machine learning (ML). However, as operational demands have increased in scope and complexity it has become clear that cloud-based AI has its limitations. There is a critical need to move AI much closer to the assets and processes that are being managed. This advanced technology is edge computing.
What is Edge Computing and how does it relate to AI?
Edge computing refers to the methodology of bringing the data processing closer to the data source, either on the device itself or a server close to the device. Rather than sending a device’s data to a data center or cloud, the edge architecture allows the data to be processed at or near the source.
Edge computing incorporates sensors attached to the targeted hardware or device. The sensors collect the data dictated by the AI algorithms and process that data on the spot. Processing and doing computational analysis on data in real-time enables the support and advancement of real-time applications, especially applications that require instant response time. According to Gartner, in 2018, only 10% of business data was produced and processed “at the edge.” By 2025, that number is estimated to reach 75%.
So, what is Edge AI?
Edge Artificial Intelligence is the same fundamental concept as edge computing. Edge AI technology refers to when the AI’s algorithms are generated and processed locally on the individual devices or machines. This revolutionary method of performing AI on the edge resolves the inability to process data and carry out AI models in real-time. Implementing AI on the edge is crucial for industries that require instant response times; namely, automotive, energy, manufacturing, telecom, and medical.
Here are the main elements of how this trailblazing approach of performing AI on the edge helps increase business performance and potential breakthroughs in technology.
Business Benefits of Edge AI
Security: Edge AI addresses the security issues that arise within a typical cloud platform. The reason is straightforward; AI edge computing operations are executed locally on or near a device, which means prominent data can be processed on the edge instead of sending it to the cloud. Fewer data in the cloud decreases the chances of an online cyber-attack, translating to better security for assets and the data they produce. Recently, many IoT (Internet of Things) systems have implemented successful hybrid solutions, utilizing the cloud for considerably large-sized applications while leveraging edge computing for processing the most salient information locally. Therefore, sensitive information doesn’t need to go through a network, foregoing the risk of being breached.
AI on the edge improves the connectivity of the data. Because the critical data is processed locally, the security and connectivity of the information are more reliable. While a step forward, the drawback of the hybrid solution method is that AI models are developed in the cloud and then pushed to the edge devices. Every edge device receives the same model, thus there is no device personalization to allow for contextualization that reflects the devices unique environment.
Latency/Speed: Edge computing improves the latency of AI applications. Latency is defined as the time it takes to transfer data between its original source and its destination. In cloud AI, data is sent from the source to the cloud and back to the source, which requires large amounts of bandwidth and cloud storage. This method can cause a delay in a device’s response time. Conversely, Edge AI brings computing to the device itself, enabling accelerated information processing and real-time analytics. For example, a slight difference in latency delaying the response time of an autonomous vehicle could be the difference between life or death.
Operational Excellence: Operational excellence refers to the ability to collect massive volumes of raw data about a manufacturing environment at the edge, analyzing it, and figuring out where improvements or changes are needed. Edge AI enables operational excellence by making real-time changes to improve the output in the manufacturing environment. Embedded AI at the edge can identify the bottleneck that is causing the entire facility to slow down.
Edge AI deployment increases the OEE (overall equipment effectiveness) via embedding and training the AI models required to support an effective predictive maintenance program. Edge AI technology reduces costs and increases the productivity of an operation through the advanced data analysis and ML algorithms that support continuous improvement solutions, for example, reduction in variability within the performance of an asset or a process.
Predictive Maintenance: Predictive maintenance is the process of using data analysis to identify anomalies in processes and potential faults in equipment so that future problems can be identified and a solved ahead of time, thereby preventing an operational disruption. Predictive capabilities require advanced data processing due to the extensive number of sensors collecting data from various sources.
Edge AI technology empowers predictive maintenance by analyzing and collecting the data locally instead of sending it to the cloud. Then, after the data has been analyzed on the edge, the analysis results are sent to the cloud. By processing and analyzing the data locally, companies save money on the cost of cloud computing and increase the speed of their audits.
Predictive maintenance brings tangible operational advantages to any enterprise that is asset and process intensive. Those advantages would include:
- Maximizing the lifespan of equipment: When sensors detect anomalies, Edge AI can fix the problem before it becomes irreversible while at the same time reducing MTTR (mean time to recover or restore).
- Improved production and product quality: The improvement of a business’ operational efficiency directly increases the productivity and grade of its work.
- Maximizing ROI: Predictive analytics enables improvement of operations, so businesses don’t leave any value left on the table for what they incur in their operating costs. According to data from the U.S. Department of Energy, deploying a predictive maintenance solution could produce an estimated 30% reduction in maintenance costs, a 75% decrease in breakdowns, and a 45% reduction in downtime.