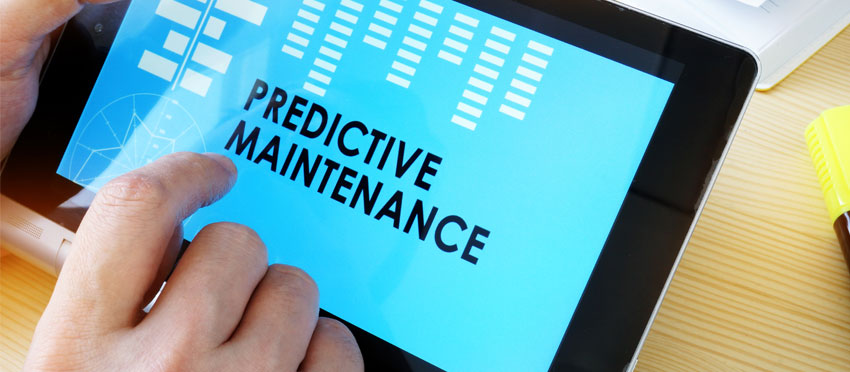
28 Oct An Introduction to Predictive Maintenance
Industry 4.0 initiatives continue to gain momentum across virtually every industrial and manufacturing segment. This fourth industrial revolution is built upon three primary technological advancements: Internet of Things (IoT), Big Data, and Edge Computing. As the global market and adoption of IoT devices continues to grow, many operations are turning to edge technology because of its relevant advantages over legacy cloud solutions. One key advantage is real-time predictive maintenance. In an edge-based predictive analytics solution, AI is combined with business intelligence (BI) to analyze the operating condition of a device or machine and help predict when one should perform maintenance on that asset.
Predictive Analytics Defined
Predictive analytics refers to the methodology of using advanced analytics and statistical algorithms combined with machine learning techniques to make assumptions about future outcomes based on current and historical data patterns. Businesses use this strategy to take advantage of possible future events by using predictive modeling to make important maintenance decisions before a disruptive event can occur. A simplified explanation of how this technique works is this: data is imported from the targeted asset, that data is then synthesized and aggregated with different data sources. After a large volume of data is cleaned, the data analysis is initiated to identify trends and patterns. Finally, using machine learning techniques and artificial intelligence, the system can generate predictive models for predicting future events.
What is Predictive Maintenance?
Predictive maintenance, a subset of predictive analytics, refers to the same process of using data analysis to predict future outcomes. More specifically, this is used to identify anomalies and potential faults in assets and/or processes. Predictive maintenance is fundamental to asset-intensive industries who utilize IoT technologies to improve the performance of their assets. According to a Gartner article written in 2018, spending on IoT-enabled predictive maintenance will increase from $3.4 billion in 2018, to 12.9 billion by 2022.
Industry Implications for Predictive Maintenance:
Predictive computing has transformational implications for any industry that depends on reliable and predictable performance of their critical assets. A couple of those industries would include the following:
- Automotive
If a car is connected, either through internet connectivity or an IoT device, it can send the data captured by the sensors on the vehicle to other external devices. That data can also be transmitted back to the sensor OEMs and the auto manufacturers. The manufacturers and OEMs can then use the information to alert the owner of the car to come in for maintenance to address performance issues before expensive damage is incurred.
- Medical
An individual’s medical data is crucial to understanding the overall state of their health and wellbeing. Equally as important is the data from medical equipment that may provide life-saving support to individuals. Hospitals have started to use predictive analytics to identify anomalies in data and diagnose potential failure in critical equipment. Notifying doctors and nurses when the data indicates a potential equipment problem before it fails and stops assisting an individual may prevent unnecessary stress, equipment replacements or avoid a life-threatening situation for the patient.
- Oil and Gas
In the oil and gas industry, even a small period of unexpected downtime leads to the loss of millions of dollars. Many oil and gas companies install IoT sensors in their equipment to assess and predict the machine conditions. They use the machines’ data to determine the operating environment’s safety, increase operational efficiency, and eliminate the chance of costly downtime.
The Primary Advantages of Predictive Maintenance:
The adoption of an AI-enabled predictive maintenance solution provides several competitive advantages when compared to legacy asset maintenance processes and protocols. Those competitive advantages include:
- Lower Maintenance Costs
When the IoT sensors attached to a device or machine detect anomalies, not only is this shining a light on a developing problem, but the intelligence embedded in the hardware can fix the problem before it becomes irreversible. This reduces the chance of costly operational setbacks due to unplanned equipment failure. According to the McKinsey management consulting firm, a predictive maintenance application can reduce maintenance costs by 25%, while Deloitte Analytics Institute believes it can reduce breakdowns by 70%.
- Reduction in Downtime
According to data from the U.S. Department of Energy, a predictive maintenance solution could produce approximately a 45% reduction in downtime. AI in the device constantly monitors and analyzes the data patterns of an asset, identifying when to plan for repairs so you can schedule them in advance. Not only does this help companies avoid costly downtimes, but it also allows them to more effectively optimize their resource schedules by only ordering spare parts as needed or scheduling maintenance outside of operating hours.
- Increased Asset Lifespan
By detecting developing equipment problems, equipment can be serviced before the problem becomes irreparable. Additionally, due to the constant pattern of machine learning identifying future outcomes based on an asset’s current and historical data patterns, the AI solution prevents any significant damage from occurring, consequently increasing the overall health of connected equipment and increasing its average lifespan.
- Increased Production
The elimination of unscheduled downtimes, combined with the ability to continuously monitor a machines’ performance, can significantly improve the throughput of a manufacturing operation. The ability to predict when maintenance will be required also has a positive effect on the quality of the products being produced.
- Maximized ROI
All the data that businesses collect on their equipment allows them to make more evidence-based decisions regarding asset management. This can maximize manufacturing value by improving the OEE (overall equipment effectiveness) and increasing the production volume, all while decreasing repair or replacement costs. It is in this way that businesses are leveraging IoT-based predictive asset maintenance strategies to maximize value and minimize costs over time.
Edge AI – the Future of Predictive Maintenance
Although cloud computing can support predictive analytics solutions, businesses gain a crucial competitive advantage by improving the speed and performance of data processing through an edge computing architecture. In cloud computing, AI models are created and deployed on the cloud and data is then sent through a network to conduct analysis. Carrying out these models on a remote server comes with potential latency complications which causes delayed response times.
In lieu of depending on quality connectivity to send data from the device to the cloud through the internet, edge technology optimizes the speed and performance of predictive analytics by performing machine learning models locally. Implementing predictive solutions through embedded AI on the edge enables real-time analytics and updates, it is this advantage that is critical for industries where a slight delay in response time could lead to a catastrophe.
In addition to real-time analytics and low latency, a predictive maintenance program performed at the edge reduces data storage costs.
IoT devices and sensors collect data as often as every second, meaning these IoT powered solutions work with a great deal of data. When solutions are executed by way of cloud computing, a high volume of data needs to be transferred over the network to the cloud. While internet workload continues to grow, networking costs will increase as well. However, solutions performed on the edge analyze the data on premise in real-time, helping minimize or reduce the amount of data transferred to the cloud, which in-turn saves businesses money on cloud storage costs.